Article
by Dillon Dayton, Solutions Architect
Elevating data quality with Generative AI
In today’s world, data is the currency of innovation, and businesses are looking to Generative AI (GenAI) to solve their data issues. We’ll navigate the uncharted waters of how GenAI can mitigate the risks of poor data quality and cultivate an ecosystem of reliable, enriched data.
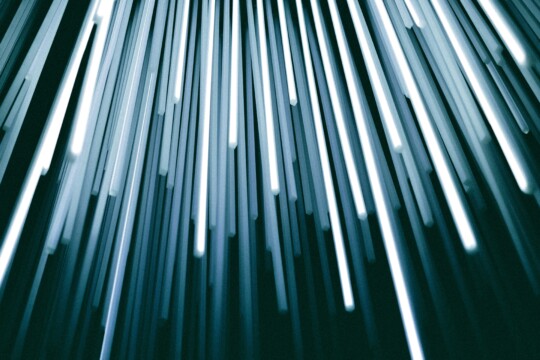
Data is the cornerstone of informed decision-making, strategic foresight, and competitive edge. However, the persistent challenge of ensuring the integrity of data quality remains an ongoing struggle. Conventional approaches fall short in today’s dynamic information landscape. Data arrives from a myriad of sources, in diverse formats, at unprecedented speeds that make traditional methods of quality assurance inadequate. This is where GenAI steps onto the stage, presenting a paradigm shift that redefines how enterprises can ensure data quality.
GenAI, a culmination of advanced machine learning and neural network technologies, holds the promise of not just identifying and rectifying data discrepancies, but also of intelligently generating high-quality data. It’s an innovation that transforms the role of AI from being a passive analyzer to an active participant in data enhancement.
Let’s explore the most common data quality issues in organizations, how generative AI can help solve or mitigate them, and look at a real-world scenario that demonstrates how generative AI can be used to drive real business value. In the pursuit of success and innovation, we recognize there are far-reaching consequences of poor data quality.
Consider the following consequences to data accuracy and integrity
Inaccurate Decision-Making
Poor data quality threatens the very bedrock of insightful and sound analyses. Business strategies, conceived and executed based on unreliable data, not only run the risk of misdirection but also incur financial losses and missed opportunities.
Lost Revenue
Inaccurate customer data, ranging from flawed contact details to incomplete purchase histories, directly undermines sales potential and chips away at customer satisfaction. The pernicious billing errors stemming from data inaccuracies manifest as revenue leakage.
Customer Dissatisfaction
Incorrect or outdated customer information begets woeful customer experiences, such as inundating patrons with irrelevant offers or out-of-touch communications. The collateral damage is substantial, including tarnished brand reputation and eroded customer loyalty.
Operational Inefficiencies
Inaccurate data ripples through business processes, disrupting supply chains with misplaced inventory data, leading to overstocking or stockouts. This operational chaos incurs lost sales, inflated costs, and disgruntled customers.
Regulatory Compliance Risks
In an era of stringent data regulations, poor data quality thrusts enterprises into a dangerous tango with non-compliance. The ramifications are dire—penalties, legal entanglements, and the enduring stain on reputation.
Reduced Trust in Data
When employees and stakeholders continually encounter errors or inconsistencies in data, trust in that data crumbles. Often due to business users creating their own version of the data creating confusion and repeated work. This overall skepticism can translate into a reluctance to rely on data for decision-making, impairing overall enterprise performance.
Strained Relationships
Poor data quality wreaks havoc on relationships with partners, suppliers, and customers. Inaccurate data sows the seeds of misunderstandings and disputes, fracturing critical alliances and forfeiting future opportunities.
Harnessing the Power of GenAI for Your Needs
There are many risks that come with continuing to live with poor data quality, but harnessing GenAI offers a wide spectrum of capabilities to help mitigate those risks. From identifying anomalies that signal data quality concerns to streamlining the arduous process of data labeling and cleansing, GenAI stands as a transformative force in an enterprise powered by data. Consider how these intelligent algorithms can reshape the way your organization approaches data quality.
- Data cleansing: Generating code or scripts for tasks such as parsing, formatting, imputing missing values, and identifying data quality issues, streamlining the data cleaning process and improving efficiency.
- Labeling and Tagging: Generative models can assist in generating initial labels, tags, and annotations, which can then be refined by human experts in governance or ML applications.
- Anomaly detection: Learn the normal patterns of data and identify anomalies or outliers that deviate from these patterns.
- Data imputation: Impute missing values in datasets. By learning patterns from existing data, these models can predict and generate plausible values for missing or incomplete data points.
- Synthetic Data generation and testing: Create synthetic datasets that mimic the distribution of real-world data. These datasets can be used for testing, validation, and training.
Real-World Scenario with Generative AI
Consider a prominent research hospital specializing in dermatology and oncology that aims to advance the accuracy of its skin cancer identification models. The hospital’s machine learning team is facing a significant challenge due to the limited availability of diverse and high-quality skin cancer images for training and testing their algorithms. Traditional methods of collecting such data are time-consuming, expensive, and can raise privacy concerns.
As previously discussed, a practical application of GenAI is to create synthetic datasets that mimic the distribution of real-world data. These datasets can be used for testing, validation, and training. For our research hospital they feel to overcome their challenges, they need to leverage this technique to create synthetic test data specifically tailored for skin cancer identification models.
Benefits:
- Enhanced Model Performance: The AI-generated synthetic data significantly increases the volume and diversity of the training dataset, resulting in improved model performance and generalization.
- Privacy and Ethical Compliance: Using synthetic data minimizes privacy concerns and ethical issues associated with using real patient images, as the synthetic data does not contain identifiable information.
- Cost-Efficiency: Creating synthetic data is cost-effective compared to the labor-intensive process of collecting and annotating real images.
- Faster Development: The hospital can expedite the development and deployment of skin cancer identification models by relying on generative AI for data augmentation.
- Rare Case Simulation: Synthetic data allows for the simulation of rare skin cancer cases, enabling the model to recognize and diagnose less common conditions accurately.
By employing generative AI to create synthetic test data, the research hospital can accelerate the advancement of skin cancer identification models, ultimately leading to more accurate and early diagnoses, improved patient outcomes, and valuable contributions to dermatological research.
Empowering Action
We believe our customers deserve to experience the power of Large Language Models (LLMs) and Generative AI within their context quickly. This is why as part of our Nortal Tark program, we are exploring how LLMs could be utilized for fundamental concepts like data quality.
Leveraging Tark we can streamline your processes, reduce errors, and ensure that your data is a true asset to your organization. Curious to discover more about Nortal Tark and how it works? Learn more about how Nortal Tark can help you harness the power of LLMs to bring value from our customer’s data on their terms.
Get in touch
Let us offer you a new perspective.